Short steps to reading a paper Part 11: On no Statistics!
This week I am going to discuss statistics. I do not have sufficient knowledge to do this in-depth, so I will keep it simple. We know that most of us shy away from reading or trying to understand statistics. Some methods are better suited to randomised clinical trials and non-randomised studies. There are also reasonably standard approaches for systematic reviews. However, I think that the following points are important when you read the statistical section of a paper:
- The authors should mention both descriptive and inferential (or analytical) statistics. Descriptive statistics outlining the sample’s nature are particularly important as they are easy to understand and. This information also allows us to decide whether the study is relevant to our practice.
- Ideally, the authors should tabulate their descriptive statistics. These tables should include demographic and clinical characteristics.
- The study team should only perform inferential testing the key outcomes only. My own view is that these should be kept relatively simple, focused and responsive to the limits of the available data. Let us look at an example:
Example 1: Two methods of overjet reduction.
In a study, we aim to compare the effectiveness of two approaches to overjet reduction (in mm) in a non-randomised study. We have approx. 60 participants: 30 each in a functional appliance group and 30 having camouflage with maxillary premolars extraction.
A simple way of comparing the means would be to use an independent t-test. This is a simple level of statistical testing called univariate analysis. But this level of statistics is not relevant to most orthodontic research projects. This is because there are many other factors (confounders) that may influence the outcome. For example, these include age, gender and baseline overjet. We would therefore account for these in an ‘adjusted’ (larger and more complicated) model. This is called multivariate analysis.
While it is tempting to include a long list of these confounders in our clever model, we need to be selective. If we are not selective, there is a danger of going on a “fishing expedition” to search for statistical significance. Specifically, there is a ‘rule of thumb’ that we need approx—10 observations for each level of each of the variables that we include.
- Ensure that the statistical approach answers the research question. This seems far, too basic to be a problem. However, it is a common issue in dental and orthodontic research. In particular, we have a tendency to ‘test against baseline’ comparing change within rather than between groups in studies that should compare interventions. Let’s look at another scenario:
Example 2: The effectiveness of two fixed appliances.
We are comparing the effectiveness of two fixed appliances (Bracket A and B) in aligning the lower dentition over 8 weeks. There is no merit in comparing the change within each group, i.e. the resolution of irregularity with Bracket A or B, in isolation, over the 8 weeks. We know that combinations of brackets and flexible wires move teeth. We are trying to decipher, which works better. Our statistical testing should reflect this and therefore centre on between-groups differences.
- Some of the studies that we undertake are split-mouth or involve clustered observations. If we assess the failure rate of attachments, for example, it is crucial to account for the likelihood that multiple failures may occur in one patient. As such, these outcomes are not independent, and this should be accounted for in the analysis.
- When you see P values, look for confidence intervals. For inferential testing, statisticians like to see the actual difference (treatment effect) between treatment groups and the 95% CIs. This gives us an idea of the magnitude of the effect and its uncertainty. In contrast, the P-value is often misused as a binary assessment of significance with an arbitrary (typically 0.05) cut-off point. A significant p-value does not always indicate clinical importance or relevance.
- The number of tests should be limited. We are all familiar with the number of tests undertaken on orthodontic data (classically concerning cephalometric outcomes). There is a risk that spurious false positive outcomes will be observed with this approach. It is, therefore, better to have a limited and pre-defined analysis plan. As the saying goes “If you torture the data long enough, it will confess to anything’.
- On a similar note to the previous, it is worth considering whether statistical analysis (meta-analysis) is the right thing to do. Let’s review another situation:
Example 3: A functional appliance study
We are comparing the effectiveness of two functional appliances (Appliance A and B) in increasing mandibular length within a systematic review. There are problems in comparing the results from prospective and retrospective studies, with the latter likely to be prone to bias and producing artificially good results. Similarly, it would be inappropriate to compare studies involving adults with adolescents. No amount of statistical testing will compensate for this lack of comparability.
Next week Kevin will look at something much more straightforward (P= 0.0001)!
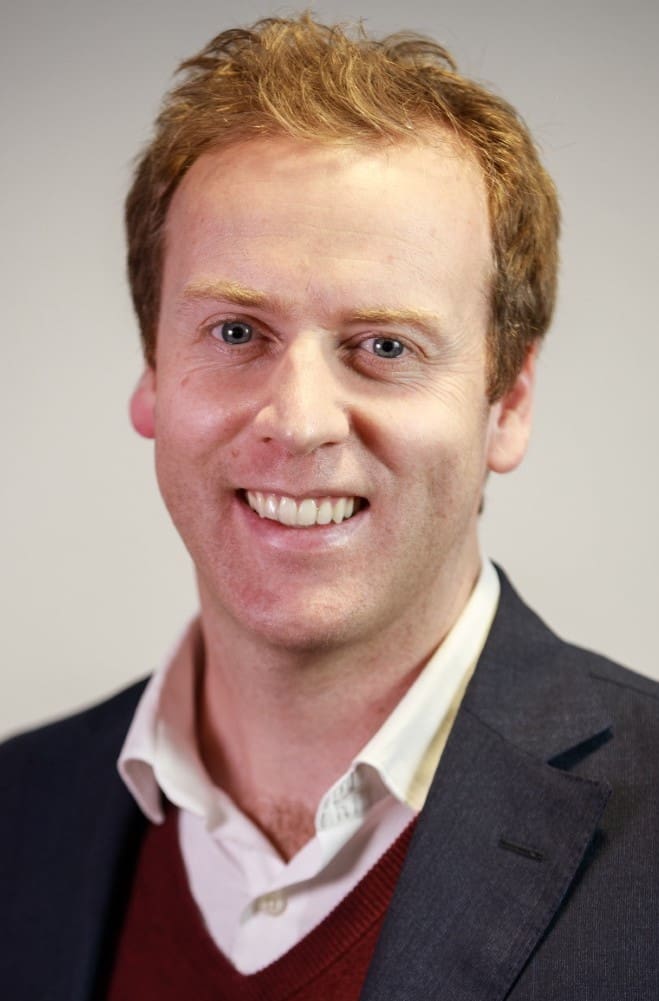
Professor of Orthodontics, Trinity College Dublin, The University of Dublin, Ireland
The silence is deafening 🙂 What is the difference between ‘statistical significance’ and ‘clinical significance’?
Thats for next week!