Can an artificial intelligence robot identify crowding and the need for extractions?
There have been significant developments in artificial intelligence over the past few years. This paper outlined how a team tested artificial intelligence models in identifying dental crowding and extraction decisions. The results of this study are remarkable. They also provide a great way forward using AI in orthodontic treatment planning.
It is well established that there is marked variation in orthodontic treatment planning decisions. This is particularly true for extracting teeth as part of treatment. Previous studies have shown that this is influenced by what orthodontists perceive when examining the patient. This is then modified by many other factors, for example, personal experience, philosophy, memories of recent results, and even their dental school. As the extraction decision is irreversible and has consequences for the progress of treatment and even the result, we should seek methods to reduce this variation.
One of these may be the use of artificial intelligence. The authors of this paper describe AI as
“Creating automated systems to perform tasks and solve problems without specific rules by learning the data as humans do”.
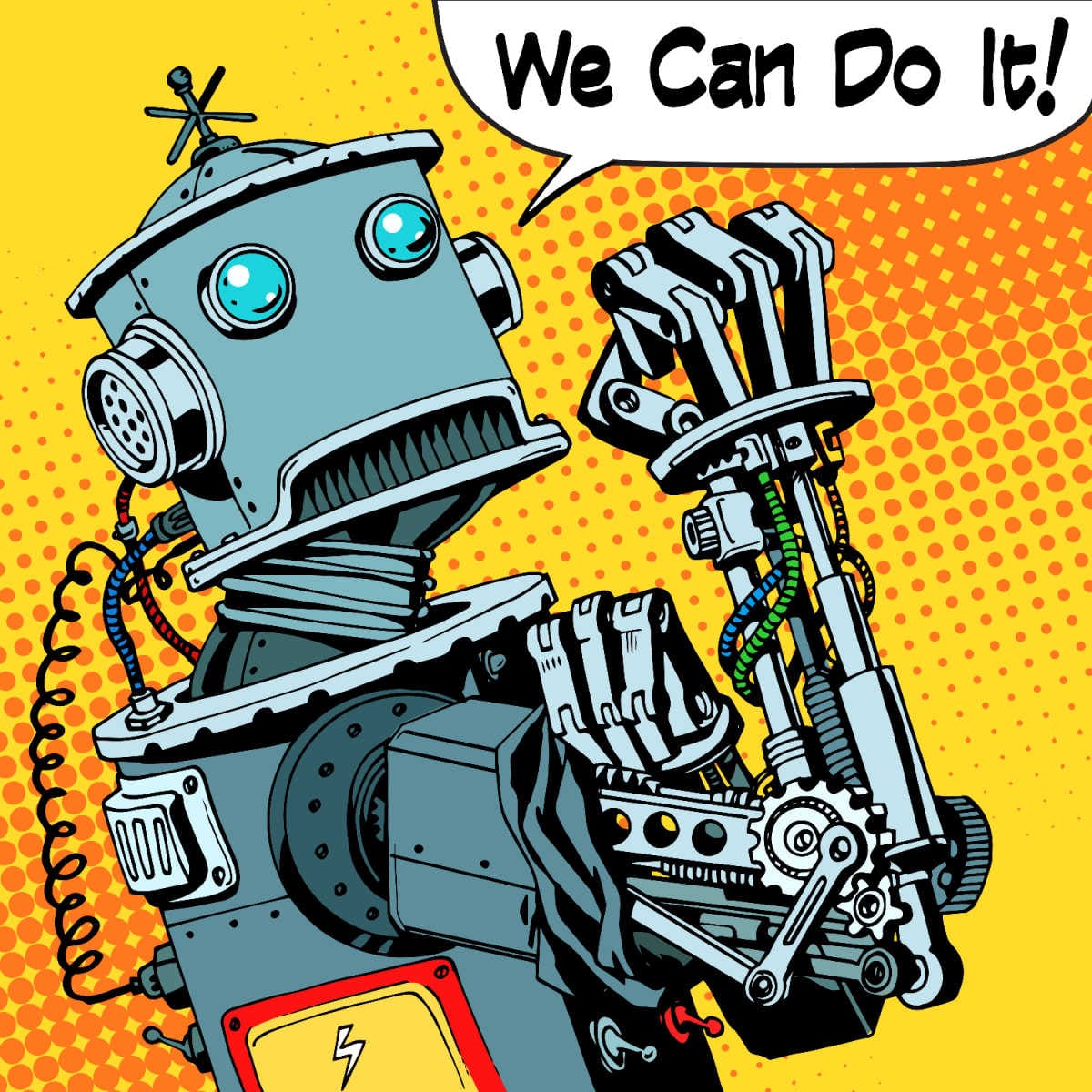
Jiho Ryu, Ye‑Hyun Kim, Tae‑Woo Kim & Seok‑Ki Jung
Nature Scientific Reports (2023) 13:5177
https://doi.org/10.1038/s41598-023-32514-7
What did they ask?
They asked
“Is it possible to set up artificial intelligence models for tooth landmark detection and tooth extraction diagnosis using feeding data from clinical intraoral photographs”?
What did they do?
This was a complex study, and I have done my best to identify their methods. Any errors in interpretation are entirely mine. They did the study in the following stages;
- They obtained 1500 maxillary and 1636 mandibular digital images from a group of patients aged approximately 26 years old.
- Two orthodontic specialists looked at the images and identified each tooth’s mesial and distal points and whether extractions were needed for orthodontic treatment.
- Then they split the images into learning and testing datasets. The test dataset included 200 maxillary and 200 mandibular photographs. The learning dataset comprised 1,300 maxillary and 1.438 mandibular photos.
- Next, they constructed artificial intelligence models to identify crowding and extraction decisions.
- They then categorised the amount of crowding by measuring the arch length discrepancy.
- Finally, they calculated the accuracy of the artificial intelligence models for the crowding categorisation and extraction decision.
What did they find?
They presented data for four artificial intelligence models. This was difficult for me to interpret. As a result, I have tried to simplify their data. These were the main findings.
They measured the accuracy of crowding categorisation with the Kappa statistic. The highest kappa for crowding was 0.73, and the lowest was 0.61. These are good levels of agreement.
When they looked at extraction decisions, they compared the decision the orthodontists took with the conclusion of the AI. They did this by constructing receiver operating characteristics (ROC). Again, these are complex to interpret. However, a simple way to do this is to look at the area under the curve (AUC). The closer this value is to 1, the greater the accuracy of the decision. The most accurate model had an AUC of 0.961, and the least was 0.934. This means that the accuracy is high.
Their overall conclusion was;
“Artificial intelligence models with proper architecture and training can substantially help clinicians in orthodontic treatment planning”.
What did I think?
This is an important paper because it shows the way forward for AI development in orthodontics. First, the level of accuracy was remarkable. It represented less variation than we see in studies examining the reproducibility of orthodontic extraction decisions.
However, before we all get excited or concerned about the implications of this paper, we need to look at the possible deficiencies closely. I thought that these were
- The study was done on photographs of the teeth only. We all know that we analyse a large amount of other information when we take extraction decisions. Nevertheless, other studies have shown that we can make decisions based on photographs only.
- The gold standard extraction decision was based on the opinion of only two orthodontists. We need input from more clinicians to make the results relevant to the real world of clinical practice.
- The patients were all in the permanent dentition and were adults.
- Finally, the study team did not consider the other features of the patient that influence extraction decisions. For example, there was no input of overjet, overbite, buccal segment relationships and facial profile.
Final comments
This will be one of many studies looking at AI in the future. Technology has a great future in helping us make the correct treatment decisions. We are on the cusp of a great opportunity for orthodontic treatment planning. I can imagine a day when data from many cases provided by a broad spectrum of orthodontists could inform IA robot’s treatment recommendations. This could be of great benefit to our patients.
Nevertheless, as with most orthodontic innovations. While there is potential for benefit, there is also the risk of abuse. I will address the pros and cons of IA in a further post.

Emeritus Professor of Orthodontics, University of Manchester, UK.
I’ve been following big names in artificial intelligence, like Stuart Russel… I have the impression that we will soon be able to compare our personal orthodontic decisions with other alternatives proposed by artificial intelligence. This will certainly allow us to revalidate or not important and irreversible decisions, such as dental extractions. In the distant future (how distant?), perhaps our personal therapeutic decisions will be considered complementary and no longer primordial. I hope that we know how to use these technological advances ethically and that the general quality of services provided by the international dental community is positively impacted.
Please Dr Kevin, keep your interest in the subject and keep us informed of new scientific work on the subject.
Thank you!
Kevin
you will to remember Chris Stephens (Bristol) developing a orthodontic planing program using iterative learning in the 1990s ; IIRC he called it Arthur
I developed a simple spreadsheet to propose extraction pattern back in the late 1990 using excel and it was on the ‘old’ learn-Ortho.com website. hhence, I’d suggest that our planning & extraction decisions are really not that complex
Surely it depends on what information you enter into the decision making process? Remember the old saying..GIGO? (garbage in..garbage out). Inputs from always extract and always never extract? Also, if the AI makes the wrong decision, who does the patient sue? Personally, I think we are a LONG way away from reliable AI “decisions”.
I would be intested to know if the two orthodontist were calibrated for the diagnosis of crowding. I couldn’t see it in the paper.